The Atmospheric Composition Modelling group focuses on enhancing the current understanding of atmospheric composition and its evolution. We synthesise weather prediction, dispersion, chemistry transport, and reduced complexity models, which can be employed to comprehend the intricate atmospheric processes influencing air quality over a region. The group utilises a variety of ground- and space-based atmospheric measurements to validate model outcomes. By harmonising diverse models and observations, we aim to provide insights for formulating effective air pollution control strategies within a specified geographical area.
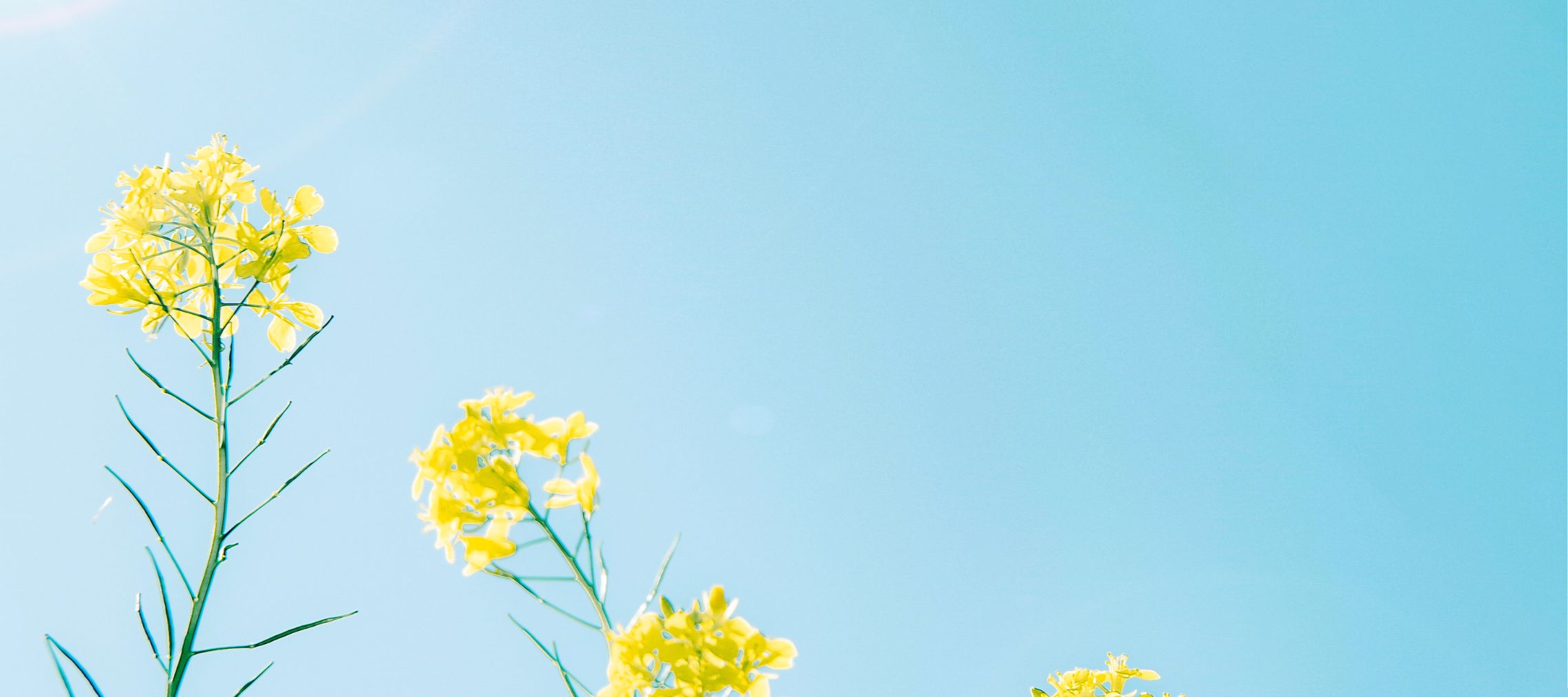
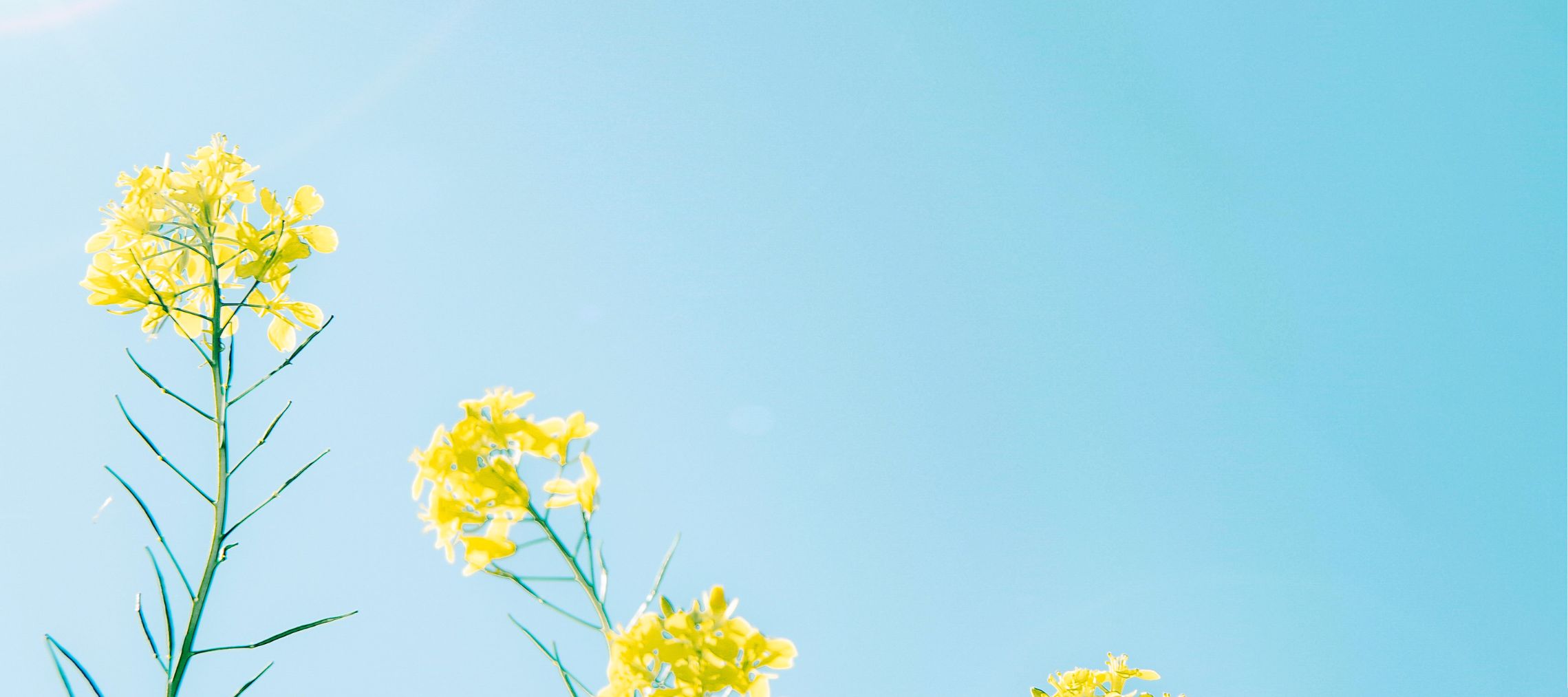
India’s need to curb black carbon emissions
At the COP26 climate talks in Glasgow in November 2021, India pledged to achieve net-zero emissions by 2070, positioning itself as a frontrunner in the race to carbon neutrality. According to the Ministry of New and Renewable Energy, India had installed a renewable energy capacity of over 180 GW by 2023 and is expected to meet its target of 500 GW by 2030.
Seasonally optimized calibrations improve low-cost sensor performance: long-term field evaluation of PurpleAir sensors in urban and rural India
Lower-cost air pollution sensors can fill critical air quality data gaps in India, which experiences very high fine particulate matter (PM2.5) air pollution but has sparse regulatory air monitoring. Challenges for low-cost PM2.5 sensors in India include high-aerosol mass concentrations and pronounced regional and seasonal gradients in aerosol composition. Here, we report on a detailed long-time performance evaluation of a popular sensor, the Purple Air PA-II, at multiple sites in India.
Multiple PM Low-Cost Sensors, Multiple Seasons’ Data, and Multiple Calibration Models
In this study, we combined state-of-the-art data modelling techniques (machine learning [ML] methods) and data from state-of-the-art low-cost particulate matter (PM) sensors (LCSs) to improve the accuracy of LCS-measured PM2.5 (PM with aerodynamic diameter less than 2.5 microns) mass concentrations. We collocated nine LCSs and a reference PM2.5 instrument for 9 months, covering all local seasons, in Bengaluru, India. Using the collocation data, we evaluated the performance of the LCSs and trained around 170 ML models to reduce the observed bias in the LCS-measured PM2.5.